E5: The Embedding Revolution w/ Anton Troynikov
PLUS: AI Doomerism, Nathan's second master thread, notable quotes and full transcript!
Hey Cogs,
Today we’ll discuss:
AI Doomerism and why we’re not all going to die
Our co-host Nathan Labenz’s recent mega thread about Open AI’s pricing and what it means for you
Notable quotes and a fully searchable transcript from our interview with Chroma’s Anton Troynikov
A look ahead and “homework” for Episode 6
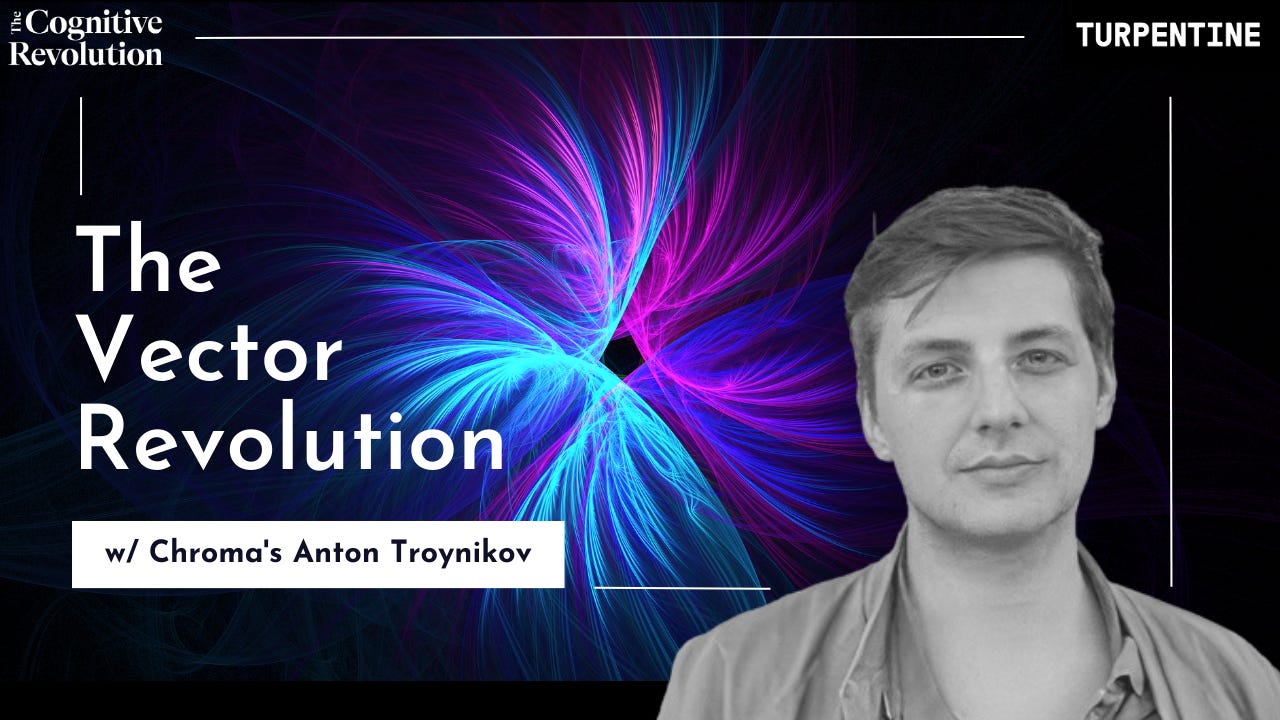
OpenAI Price Drop: [Another] Megathread
On March 2, Nathan dropped another megathread on OpenAI’s pricing. He explains the price drop on Open AI’s ChatGPT API, how it happened and what it means.

Full article here.
We’re not all going to die, but there are dangers
Anton does not believe that humanity will die due to the dangers of AI, as every argument in that direction requires a system of incomprehensible power. He is more worried about the destabilizing effects of new media technologies, which can lead to violence and totalitarianism. Anton mentions the printing press, radio, and television as examples of destabilizing media technologies that have caused violence and totalitarianism in the past. He is concerned about AI being used to create individually targeted propaganda or to allow people without the necessary knowledge to do dangerous things. He believes that we need to be vigilant about these risks, even though he does not think that humanity will necessarily die as a result.

Notable Quotes from Episode 5 with Anton Troynikov
“Chroma uses a vector database as a technology, but to think Chroma really is a platform for basically embedding knowledge that your machine learning-enabled applications can use. You can think of us as the thing that acts as the storage layer for applications that use large language models in the loop. So we're much more than a vector store.”
“While we were building this product the affordances of the existing vector stores on the market don't really suit this use case very well at all. And we actually built this product in-house and we were using it in our own product experiments for a while. Chroma basically started on the principle that you can understand the model's behavior based on how it sees its data basically. And, embedding is a representation, is a model's representation of its input data.”
“We evaluated literally everything else in the market and it just didn't fit our needs of like rapid development, rapid experimentation. It was either too hard to deploy and keep running because it was really designed for heavyweight workflows from day one, or it didn't really provide the kinda price-performance point that we needed. Or it was just, frankly, too complex.”
“And I think those models are gonna be around and continue to be there because they're so great and are almost like utilities really. But on the other side, I think what we're gonna see is these smaller, leaner models, which are actually trained to find and compose knowledge in response to queries rather than store knowledge in their own weights. And we've seen early signs of that.”
“It's really an interesting time to be working. I think that there are also just untapped veins of research here. The reason we noticed this very early on, even before we started Chroma, is that the incentives between, let's say academic ML/AI research and industrial-academic AI research, and I don't mean universities versus industrial research labs cause they're mostly doing similar work now. I mean more like the sort of work that production machine learning deployments are interested in versus the sort of work that maybe pushes you towards AGI, is fundamentally different. One of those big differences is in academic research, there are accepted community benchmarks and your aim as a scientist is to demonstrate the performance of your model on these accepted benchmarks, right? But the benchmarks are static. Whereas in the real world, the data is always changing. And questions should I train my model? What do I train it on? Is it working better or worse? Monitoring it…measuring it are much more salience than demonstrating performance on benchmarks. We founded Chroma with that observation in mind.”
“When we talk about the similarity in machine learning, it's not about perceptual human similarity. It's about how the model perceives the difference between two objects. This is important to note because the influence of one object on another is not necessarily the same as how an image might influence a human artist. The model's interpretation is very mechanical and raw - it focuses on similarities between vectors. Even if two images look quite different to a human, their vectors might be very similar to the model. Machine learning models don't encode meaning, they pursue an objective, which can produce surprising results. This is because models might focus on different things than a human would when interpreting an image.”
“In the early days of aviation, we had an intuition about what made airplanes fly, but we didn't have engineering principles or tools to develop them. Despite being wrong about how the Wright flyer worked, it still flew, and this led to a Cambrian explosion of different types of aircraft. Similarly, with machine learning today, we have a lot of intuitions and some papers make assertions about how things work, but we're still in an era of experimentation. It doesn’t matter if our intuition is wrong, as long as we can demonstrate that it works.”
“One of my favorite things about Kurtzweil is I went on the website not long ago and it was like the 10th anniversary of the singularity is near. And that just, made me laugh… So look, I don’t think we're gonna die. I have yet to see a convincing argument that we're gonna die. Every argument that I've seen in that direction requires some sort of system of basically incalculable and definitionally incomprehensible power, which if you're gonna invoke magic, you might as well just do that at the start.”
“What worries me is that we’re seeing cycles in history repeat themselves. When new technologies like the printing press, the radio, and television were invented, they significantly destabilized society and often led to violent periods. The 30 years’ war is a good example of this. It was partially triggered by the printing press because Martin Luther was able to spread his ideas quickly throughout Europe. This caused players in power to attach themselves to the movement, leading to a very violent period. We need to be aware of the potentially destabilizing effects of new technologies and take steps to mitigate them.”
“I don't think there has ever been a time in history where someone said, ‘Get ready, we're going to press this button and everything’s going to change.’ However, I believe this speaks to our resilience as a species. Every time we’ve faced significant change, we've come through it. Some people view the current situation as a unique risk, but the actual risks we face are similar to those we have faced before. Therefore, I do not say that we will be fine because we always have been before. Instead, I believe we will be fine because the actual risks we face are similar to risks we have faced before.”
“If we end up living in a society where somebody makes me implant a computer in my head, or it’s like socially unacceptable to not implant a computer in my head, I’m going into the woods…I’ve seen how software is written. I don’t want it anywhere near my brain.”
Thank you Omneky for sponsoring The Cognitive Revolution. Omneky is an omnichannel creative generation platform that lets you launch hundreds of thousands of ad iterations that actually work, customized across all platforms, with a click of a button. Omneky combines generative AI and real-time advertising data, to generate personalized experiences at scale.
Transcript
The full transcript is published at the cognitiverevolution.ai. For those that would like to save a version locally, please find the transcript attached.
On the next episode of The Cognitive Revolution, we have Junnan Li and Dongxu Li of BLIP and BLIP2. The episode will drop tomorrow Thursday, March 9th.
If you want to open a few browser tabs in advance of our next episode to prepare:
Original BLIP demo
BLIP 2
🔥BLIP-2🔥 demo is live! Come play with LLMs that can understand images and share your examples! huggingface.co/spaces/Salesfo… Project page: github.com/salesforce/LAV… BLIP-2 knows mass–energy equivalence! More examples in the 🧵BLIP is considered the 18th most highly cited paper in 2022
Image captioning comparison tool
Until next time.
"Anton does not believe that humanity will die due to the dangers of AI, as every argument in that direction requires a system of incomprehensible power."
Our usual experience with computers is that they become more powerful over time. With a doubling time of x years, 10x years gives you an AI a thousand times as powerful as the first. If you wait long enough, a billion times as powerful.
So, sooner or later, you will have an AI of incomprehensible power.